Facebook, Uber, Talk Talk, Equifax, Yahoo and Carphone Warehouse combined were fined £2.5m for breaching security around our personal data. That was before GDPR. Life post-GDPR has shifted from a non-event over the past few months to a sobering, jaw-dropping wake-up call this week - it has just got interesting. Did the UK Regulator put the decimal point in the wrong place? I read that British Airways is to be fined £183m (1.5% of worldwide turnover) and then Marriott £99m. We now need to move away from the slideware, policies, processes and paperwork and start taking practical measures on protecting our sensitive data. This article outlines what customers are asking for in the area of post-GDPR Data Management and how it is becoming a reality.
Make Money, Save Money, Reduce Risk
The sales teams tell me that there are three phrases which will attract the attention of a Customer: How do I…make money, save money and reduce the risk of fines and reputational loss from failing to comply with regulations. If Facebook can generate revenue approaching one billion dollars a week from their data, why can’t my company monetise its data? (Figure 1)
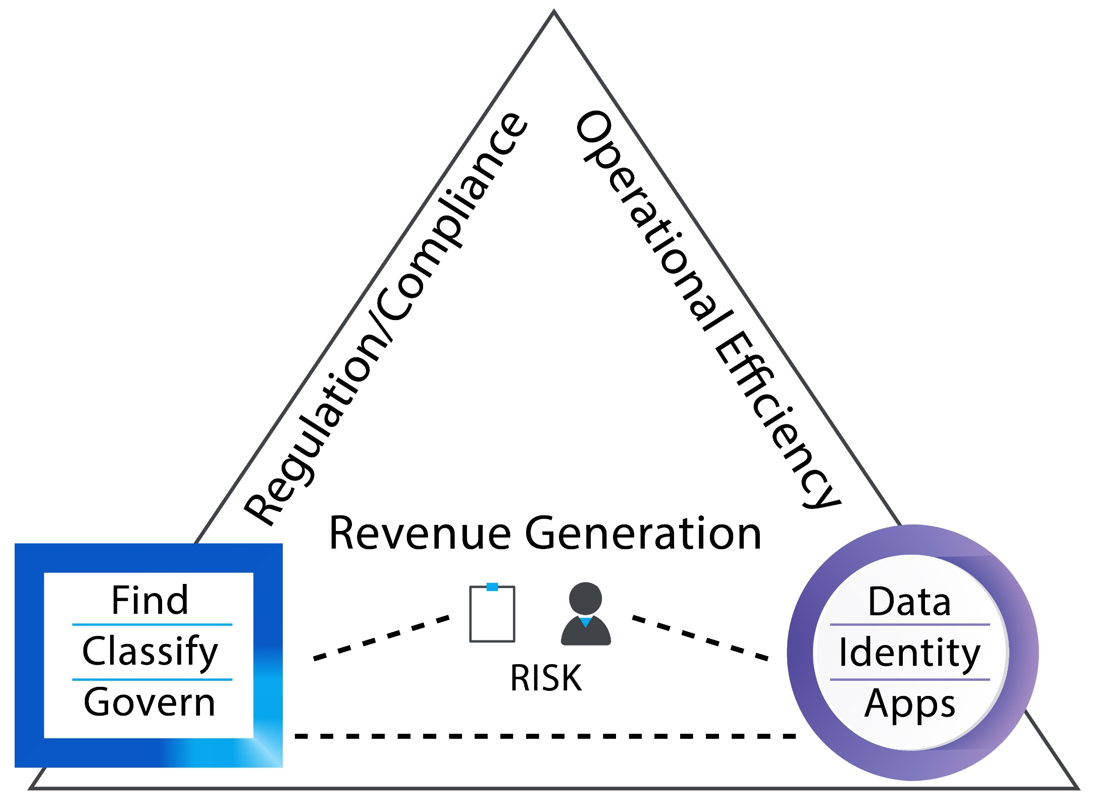
As for the objective of generating revenue, the big data story of dynamic cross-repository analytics can be told. The objective of cost saving can be addressed by reducing the amount of data; by making data more readily accessible; and reducing the time spent on finding the right data by reducing the risk of re-inventing the wheel due to failing corporate memory.
Finally, to reduce risk - reduce your data volumes; but also add a layer of security and data management.
Most of you reading this will already know these areas well in theory; but the questions I get are more practical, ‘how’ to bring this to fruition and what are other companies doing to address these issues. I hope to shed light on this area and move away from the typical marketing slideware you usually see around the ‘why’.
Is Data really important?
I recently wrote a thesis on Big Data; my lecturer insisted that I include opposing positions, a Data-driven company and a complete contrast, such as a farmer. Here, the point was to emphasise that the big data story may not be everywhere.
I later regretted that statement, because at a local Farm Open Day I discovered just how much data they collect: each animal and piece of machinery is monitored for costs and output (even tractors use cruise control with GPS for optimal harvesting!). Data, data, data; every business, everywhere. Data is money; data is cost; and data is risk.
Data has costs
Data stored in databases is known as structured data. Data in files is called unstructured data and includes images, sound and standard documentation. It costs money to store data, not only for disk space (upward of $120 a year/Gb [3]), but also in hardware, software, staff and licenses (also, maybe, in GDPR fines?).
Regardless, many organisations still see this cost as manageable and keep stockpiling data without consideration as to what data they keep and to what purpose.
I often suspect that this is because the cost and risk of data is distributed across many business functions and not easy to un-entangle.
However, now that legislation around data management has come into force, many businesses have to start to address these issues and are increasingly questioning if stockpiling data in ‘cheap’ storage is a good idea. In effect, the more data you have, the more you need to maintain in support of new regulation, the higher the cost of eDiscovery in a litigation scenario, the bigger the costs of backups, and so on.
In addition, the move to the Cloud is also making companies think about what they want to store ‘in the cupboard’ and what to put ‘in the bin’.
Data has value
The value of data comes from finding ways to monetise it and predictive analytics is the key to achieving this. The company is able, using this information, to predict target audiences for marketing, advertising, product development, product sales and so on. Seeing the future in a crystal ball and being there before the competition is the Holy Grail of business.
Data lakes have been around for a long time now and Data Scientist as a job title is becoming common: Davenport and Patil (2012) in the Harvard Business Review 4 and even the British Broadcasting Company (BBC 2019)1 suggest that Data Science is now ‘sexy’.
Data has risk
Managers for Compliance, Governance and Information Security are all too aware that a data loss, or breach, can have a big impact on the Company balance sheet. Clear-cut fines relating to GDPR are obvious, but there remain further implications, particularly around Shareholder value, with Paul Bischoff’s (2018) research report2 stating:
“In the long term, breached companies underperformed the market. After 1 year, Share price grew 8.53% on average, but under-performed the NASDAQ by -3.7%. After 2 years, average share price rose 17.78%, but under-performed the NASDAQ by -11.35%. And after three years, average share price is up by 28.71% but down against the NASDAQ by -15.58%.”
Data Management in the Enterprise
So the need is to make money from analytics, save money by reducing costs and keep data risk as low as possible – how? I have broken this down into a few simple steps and provided anecdotal customer evidence for each:
Step 1: Don’t panic, you cannot do it all!
Companies have very large data repositories, from email stores to database warehouses. The size of production data (not archived) is still measured in hundreds of Terabytes for many Customers, but we also deal with companies with data quantities measured in Petabytes.
The reality is you cannot manage all your data for costs and risk. Capabilities in software to create parallel threads of work can speed things up, but at the end of the day the size of such systems becomes very complex and costly. The only thing to do is to accept that you need to divide the data-estate into prioritised pieces and actively delete data with no business value. This approach is pragmatic and been used by a number of our Customers.
Step 2: Decide what data is important to you
Companies, if left unchecked, will naturally stockpile and create data silos. You can think of this as analogous to the second law of thermodynamics; if left unchecked the entropy of data will increase over time.
One telecoms company I visited had hundreds of old applications accumulated though years of Acquisition and Merger activity. These applications, from CRM to Financials, were kept switched on ‘just-in-case’ and no one would take responsibility for their closure – they wanted our archiving solution to enable them to close down many of these systems for good, to save money.
Data discovery is also a key Customer requirement we come across. Many companies do not have knowledge of the content of their applications – especially for Personal Data in light of GDPR. To this end, momentum has grown during the past years for software tooling with capabilities to Discover content in databases and files so that they can comply with needs such as Subject Access Requests.
A final note here is that we have seen many customers take months to decide what data needs discovering in the first place. It is crucial to plan in plenty of time to decide on exactly what data is important to you! (Remember this is not only a personal data exercise, it could be related to other areas including intellectual property, other regulation or litigation).
Step 3: The Company Data Environment
Companies will commonly tackle the ‘discovery’ phase without any real thought as to what to do next. They see this as a fast win. However, this is a problem; analysing the data in the estate takes time and you might have to start again if you missed something you had not planned for.
I have regularly advised companies to think twice before setting out on the discovery phase, unless they are absolutely sure they have an end game-plan in place. You need to answer the question ‘How will this discovered data exist in your Enterprise going forward?’
Personally, for unstructured data, I like to think about a Data Continuum within the Enterprise - and all data should fit into it somewhere. Here, think about how a file is created on a laptop and think about where it should end its life. During this life-cycle, a file should go through a continuum where, as it gets older, it is filtered for company relevance as a Business Record, finalised, archived and then protected until being defensibly destroyed. (Figure 2)
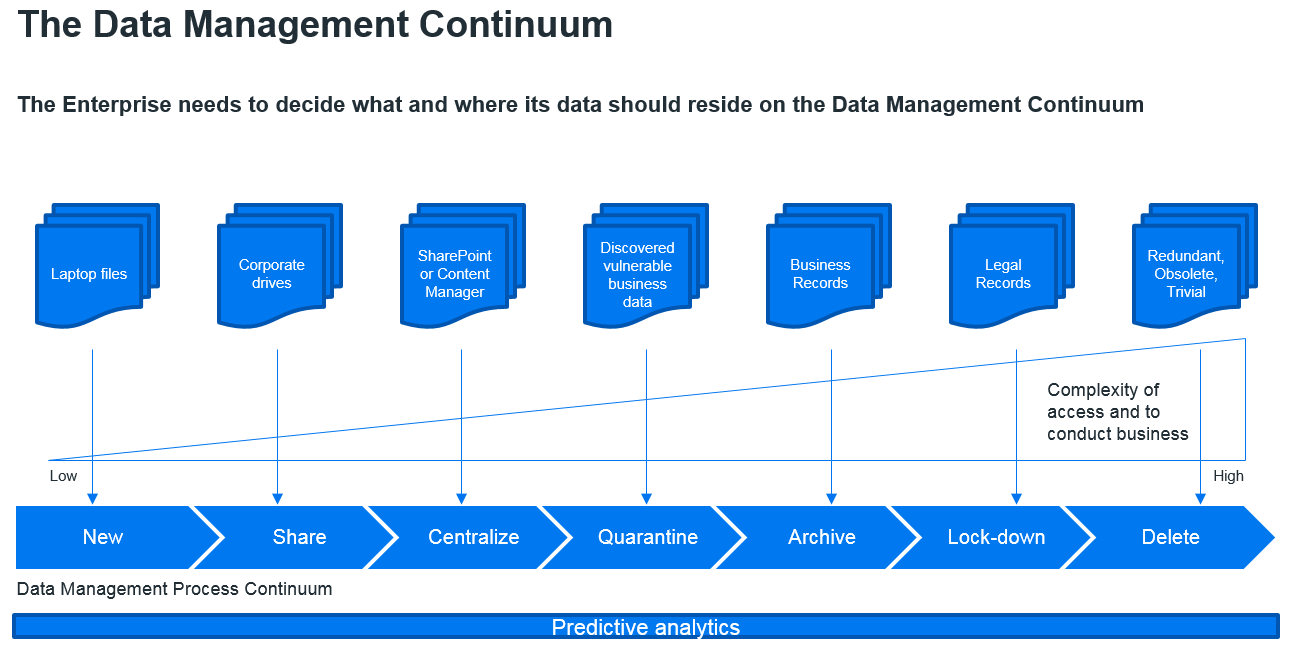
Step 4: Redundant Obsolete Trivial (ROT) Analysis
When you delete Redundant (duplicate), Obsolete (old) and Trivial data you can save yourself a huge amount of money in storage costs. If you are thinking about discovery, do a ROT analysis first to reduce the amount of data you need to analyse. If you are moving into the ‘Cloud’, do this first to save on storage costs. If you are thinking about your company’s data breach risk-profile, then do this first.
In most cases, when managing your data, use ROT analysis along with deletions first. A common rule of thumb is that organisations typically (seen over the whole organisation) can reduce the data they keep by up to 30%. This translate into 30% less storage space, 30% backup infrastructure, 30% storage maintenance (electricity, monitoring etc) and so on.
One of our Customers approached me one day asking for some way to find file duplicates. The reason being that they had implemented a SharePoint storage policy where everyone now had to store important files only in SharePoint. However, most end-users had simply copied all files from the file-share into SharePoint, but not deleted the originals. Simple ROT analysis and deletions could have taken their pain away.
I am also told by my American counterparts that many of their customers are very happy to out-right delete ASAP; just as soon as they no longer need to keep the file (not forgetting their legal obligations). The driver for this approach is to minimise the risk of lawsuits, where in the past old CEO emails have caused companies a lot of legal trouble. (Figure 3)
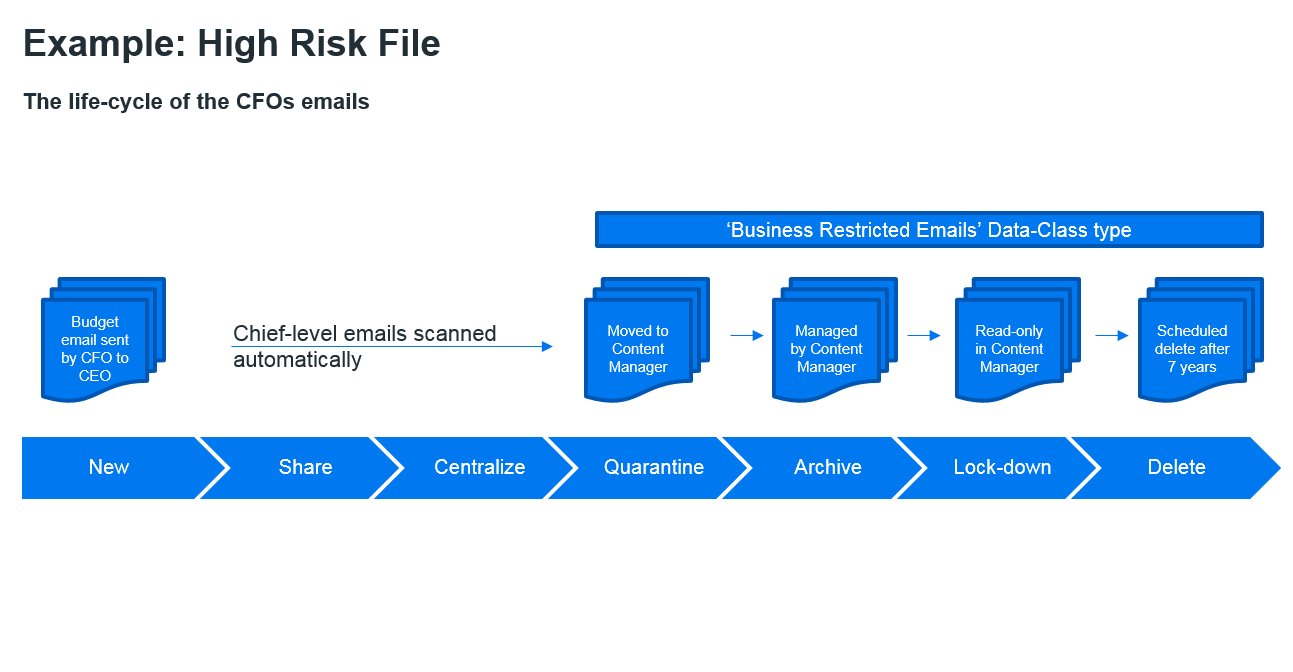
Step 5: Data Life Cycle
Over the last 3-years we have seen a growing trend for the need to track data in-place within the corporate estate. A simple example might be the need to track a banking customer and the grouping of all their transactions and related activities.
This grouping of data could be seen as an ‘entity cluster’ referenced by the Account Number - so we are not tracking at row-level in databases we are tracking groups of rows on groups of tables. Why? Because it supports GDPR requirements e.g. Subject-Access-Requests and Right-to-be-Forgotten.
The same goes for unstructured data, here we see customers carrying out discovery predominantly on their file-shares, Exchange and SharePoint. Here, files are also grouped, tagged and categorised, and then set up for automated scheduled management such as centralisation, archiving and deletion.
This capability will also be a key element in PSD2/Open Banking where organisations will need to be able to share data across open APIs. In doing so they must first be able to aggregate all relevant data across their various internal systems.
Step 6: Security
Security of data is a very large part of the story to reduce the risk surrounding data. In short, find what is important then protect it! Protect the access, protect the application and protect the content.
Each area requires careful consideration and is too large a topic for this article. Suffice to say, Micro Focus has software solutions with capabilities to manage, correct, monitor, audit, encrypt, alert and detect unusual activity on data in real-time.
Step 7: Predictive analytics
Predictive analytics is another way of monetising your data. This is a multifaceted and complex area and deserves an article in its own right, but let me just cover the key points. This can be demonstrated when you find and manage the data important to you and use it for data mining.
I can tell you that Micro Focus has some ‘Formula One’ quality software which can detect, copy, collate, aggregate, scrub, sanitise and then pass real-time information to a Data Scientist! The more accurate the managed data the better, so ROT analysis helps this position as does having a clear vision where analytics should fit against your Data Management Continuum model.
Putting it all together
From the steps above you will see that you need to decide what data is important to your company, isolate it, protect it and analyse it for value.
The diagram in figure 4 summarises the activities above and provides you an insight into the Use Cases involved - we call this the Business Information Supply Chain, shaped like a London Tube Map with different coloured lines supporting different threads of work.
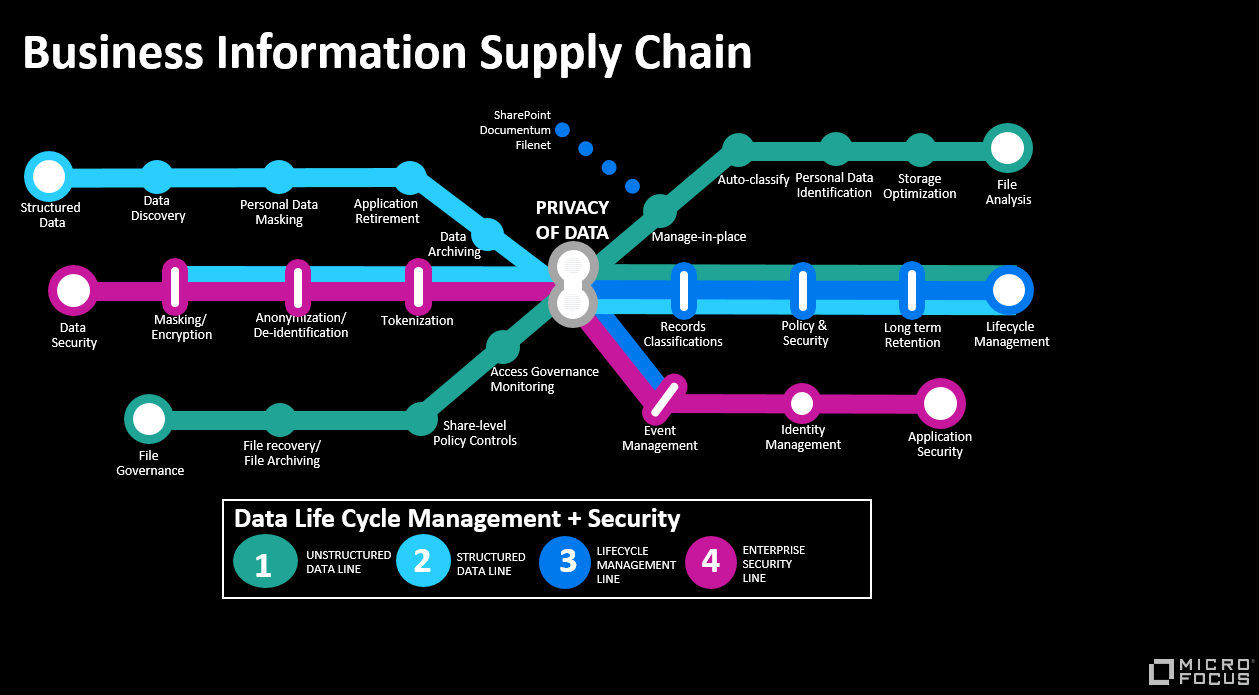
Micro Focus ControlPoint
Micro Focus ControlPoint has been discussed in previous issues. Here, I want to briefly touch on how it can support some of the capabilities discussed above.
ControlPoint is one of over 20 products which Micro Focus sells in support of Security, Risk and Governance of Data. It is focused on unstructured data which is the Dark Green line on our Business Information Supply Chain. ControlPoint can analyse for ROT and categorise and take action on file content and metadata (Figure 5).
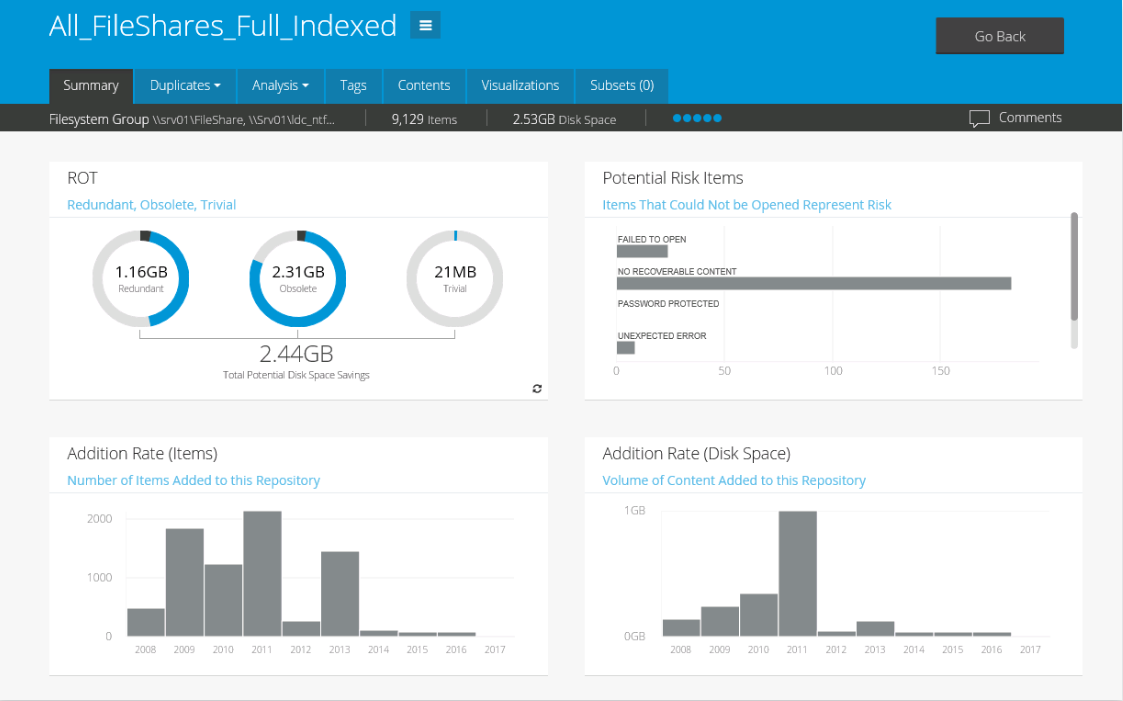
Most importantly it is the Gartner Leader of Leaders because, in addition to the above, it uses heuristics to find files of certain types and does not only rely on single-word search content alone. In effect the end-user can say ‘Show me all the files which are similar to these examples’. (Figure 6).
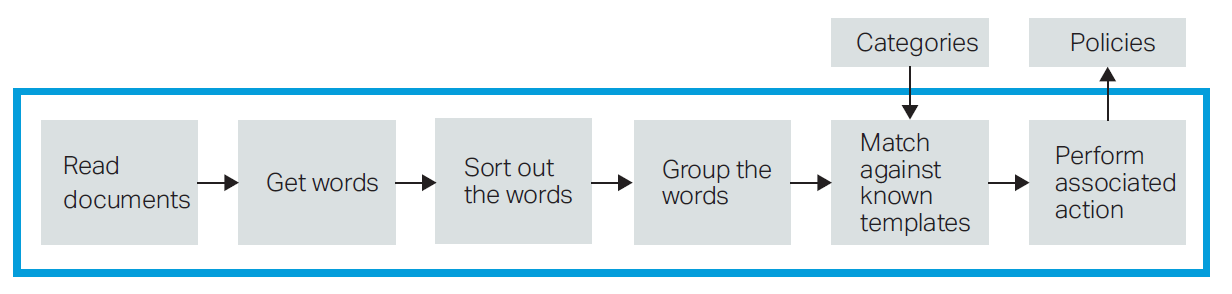
ControlPoint gives you the flexibility to perform specific actions on files, in-situ. These in-place capabilities simplify the data management by not only automatically discovering within files, but also allowing categorisation, tagging and subsequent life-cycle policy. (Figures 7 and 8).
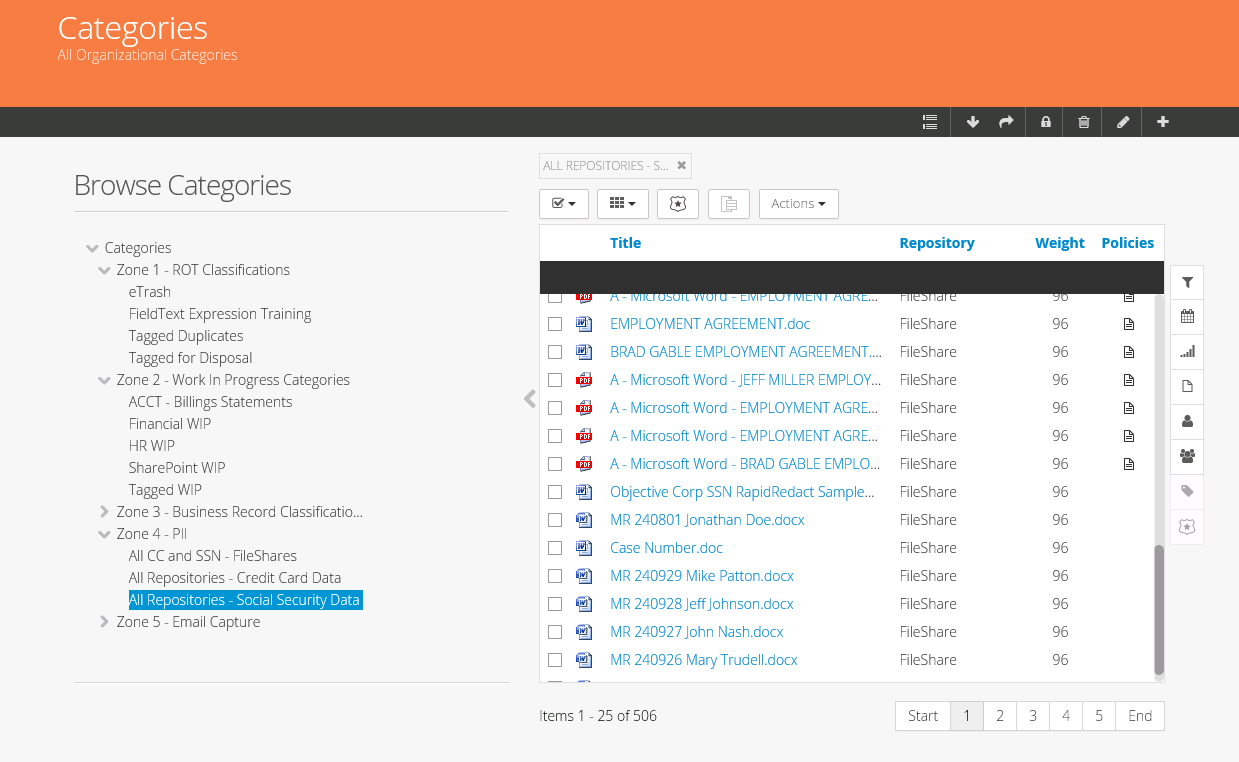
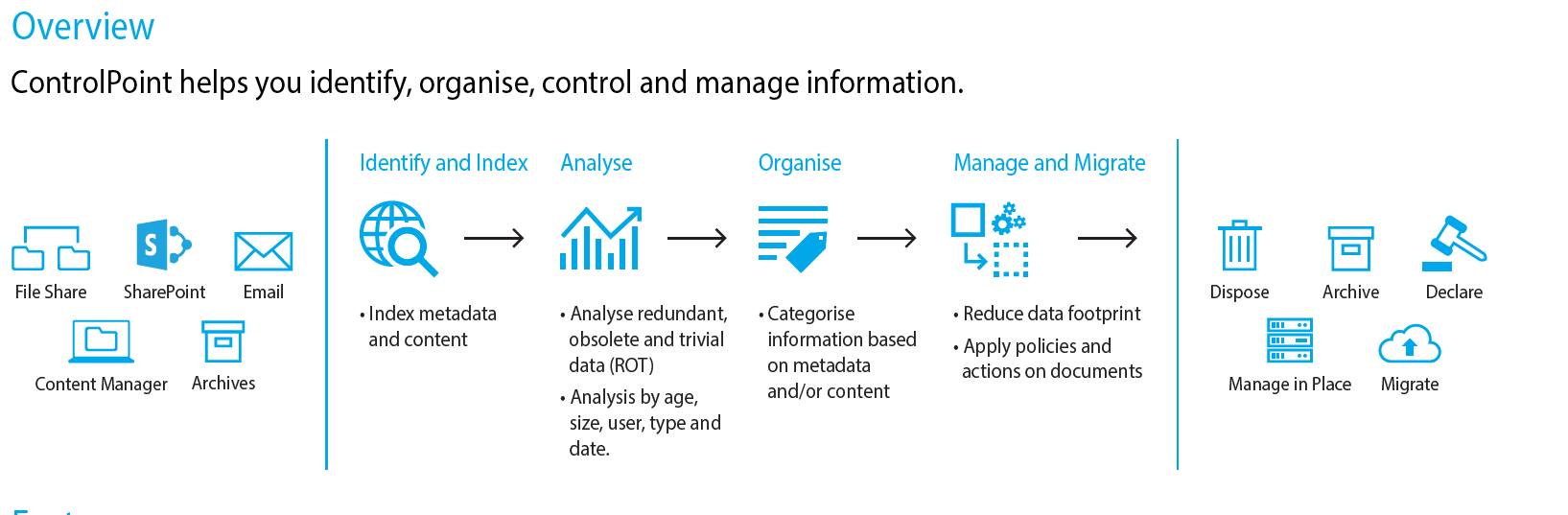
Summary
In summary, you can make money, save money and reduce the risk around data in your Company by simply swallowing the short-term pain now, but then gaining in long term benefits after. Hopefully the steps above provide a taster of how to go about getting started.
Bibliography
- BBC (2019). The Joy of Stats. https://www.bbc.co.uk/programmes/b00wgq0l
- Bischoff P (2018). Analysis: How data breaches affect stock market share prices. https://www.comparitech.com/blog/information-security/data-breach-share-price-2018/
- Mierau P (2017). How much does it cost to host 1GB of cloud storage? https://www.quora.com/How-much-does-it-cost-to-host-1GB-of-cloud-storage
- Davenport T.H. and Patil D.J. Data Scientist (2012). The sexiest job of the 21st Century. https://hbr.org/2012/10/data-scientist-the-sexiest-job-of-the-21st-century
This article was first published in OH Magazine, Issue 44, 2019.2, p10-14.